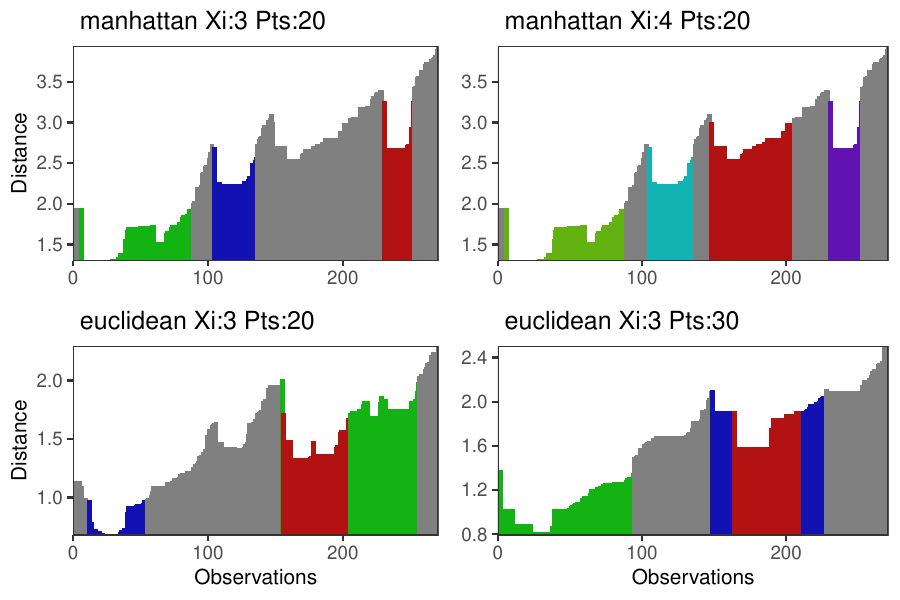
Density-based clustering methods are well adapted to the clustering of high-dimensional data and enable the discovery of core groups of various shapes despite large amounts of noise. The opticskxi R package provides a novel density-based cluster extraction method, OPTICS k-Xi, and a framework to compare k-Xi models using distance-based metrics to investigate datasets with unknown number of clusters. This article ␜rst introduces density-based algorithms with simulated datasets, then presents and evaluates the k-Xi cluster extraction method. Finally, the models comparison framework is described and experimented on 2 genetic datasets to identify groups and their discriminating features. The k-Xi algorithm is a novel OPTICS cluster extraction method that speci␜es directly the number of clusters and does not require ␜ne-tuning of the steepness parameter as the OPTICS Xi method. Combined with a framework that compares models with varying parameters, the OPTICS k-Xi method can identify groups in noisy datasets with unknown number of clusters.
Links
© All rights reserved, Thomas Charlon, 2025.
Template by Bootstrapious. Ported to Hugo by DevCows.